class: center, middle, inverse, title-slide # Challenges in
detecting evolutionary forces
in language change
using diachronic corpora ### Andres Karjus
(supervised by Kenny Smith, Richard A. Blythe, Simon Kirby)
Centre for Language Evolution, University of Edinburgh
### CLE seminar, 6.11.2018 --- class:inverse <style> .remark-slide-content { padding-top: 7px; padding-left: 25px; padding-right: 20px; padding-bottom: 30px; } body { line-height: 3em; } .mjx-chtml{ font-size: 100% !important; } .small { font-size: 50%; margin-top:0em; margin-bottom:0em} p {margin-bottom:0em} </style> # A bit of background --- # A bit of background -- - All natural languages change over time -- - Many have suggested that language change, like other evolutionary processes, involves both directed selection as well as stochastic drift .small[(Sapir1921, Jespersen1922, Andersen1987, Mcmahon1994, Croft2000, Blythe2012)] - Number of ways in which selective biases may influence language change .small[(Kirby2008, Smith2013, Enfield2014, Croft2000, Haspelmath1999, Labov2011, Mcmahon1994, Zipf1949, Baxter2006, Daoust2017; +et-al.'s )] -- - Signatures of selection should be inferable from the usage data .small[(Sindi2016, Reali2010, Bentley2008, Amato2018, Kander2017; +et-al.'s)] --- background-image: url(papershot.png) background-size: contain --- # Newberry et al. 2017, Detecting evolutionary forces in language change - _"...we quantify the strength of selection relative to stochastic drift in language evolution."_ -- - _"...time series derived from large corpora of annotated texts"_ - English verb (ir)regularization; COHA - Frequency Increment Test (FIT) -- - _"...this work provides a method for testing selective theories of language change against a null model and reveals an underappreciated role for stochasticity in language evolution."_ --- # The Frequency Increment Test (FIT) - Feder et al. 2014 .small[(from a family of tests of selection, cf. refs in paper)] - Series of relative variant frequencies `\(v_i \in (0,1)\)` at time `\(t_i\)` - Transformed into frequency increments - `\(Y_i = (v_i-v_{i-1}) / \sqrt{ 2v_{i-1}(1-v_{i-1})(t_i-t_{i-1}) }\)` -- - Rationale: under neutral evolution, the increments `\(v_i-v_{i-1}\)` are normally distributed with a mean of 0, and variance ~ `\(v_{i-1}(1-v_{i-1})(t_i-t_{i-1})\)` (inversely proportional to effective population size; when `\(0<<v_i<<1\)`; Gaussian approximation of the Wright-Fisher diffusion process) -- - Test under the null hypothesis of drift ~ test that the increments are normally distributed with a mean of 0 (e.g.: one-sample `\(t\)`-test). --- 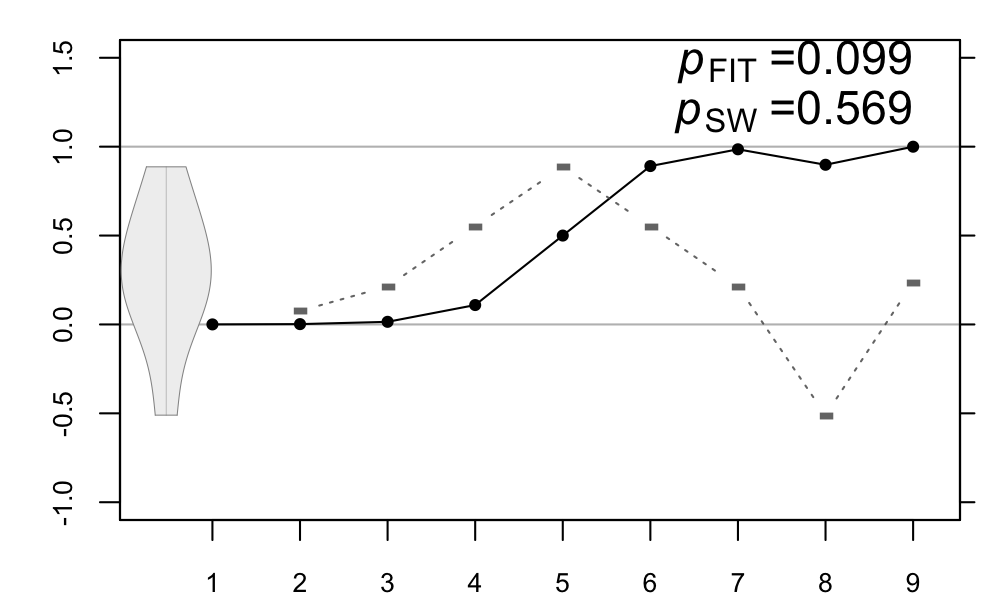<!-- --> --- # Problem: how to bin the data for time series - Microbial experiments: samples that are taken at chosen intervals and resequenced - Common approach in corpora usage: bin fixed length time segments - there is always a minimal time precision threshold (COHA: years) - but often not enough observations at fine precision - so: decades, years, days, minutes - example: daily newspaper -- - Newberry et al.: use variable width quantile binning, n(bins) = log(total frequency). Assures ~same number of occurrences per bin (but bins cover different lengths of time) --- .pull-left[
] .pull-right[
] <span style="font-weight: bold;color: #D58639"> `\(p<0.05\)` </span><br> <span style="font-weight: bold;color: #CDCD8D"> `\(p<0.2\)` </span><br> <span style="font-weight: bold;color: #C1EFFF"> `\(p>0.2\)` </span> --- class:inverse # Replication of Newberry et al. 2017 (36 verbs) --- # Replication of Newberry et al. 2017 (36 verbs) 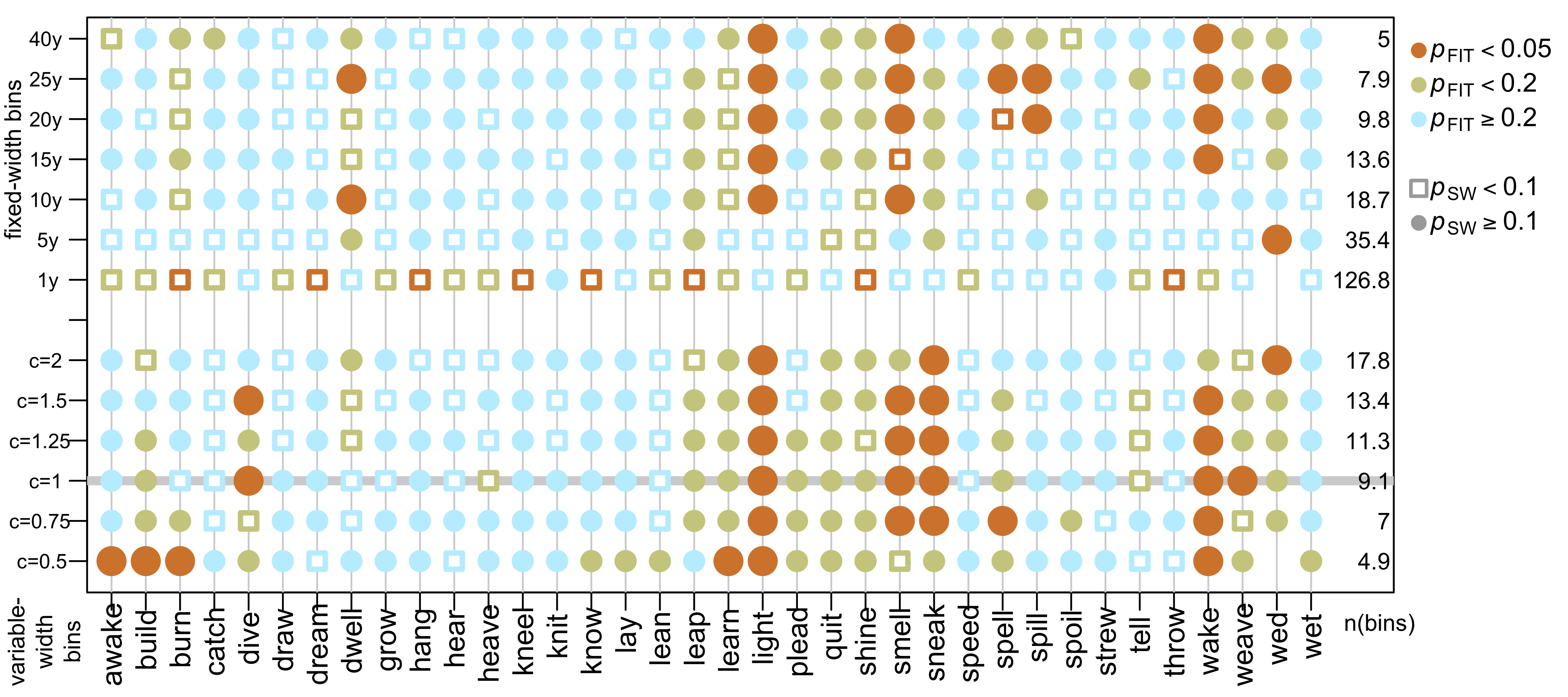<!-- --> --- # Replication of Newberry et al. 2017 (36 verbs) 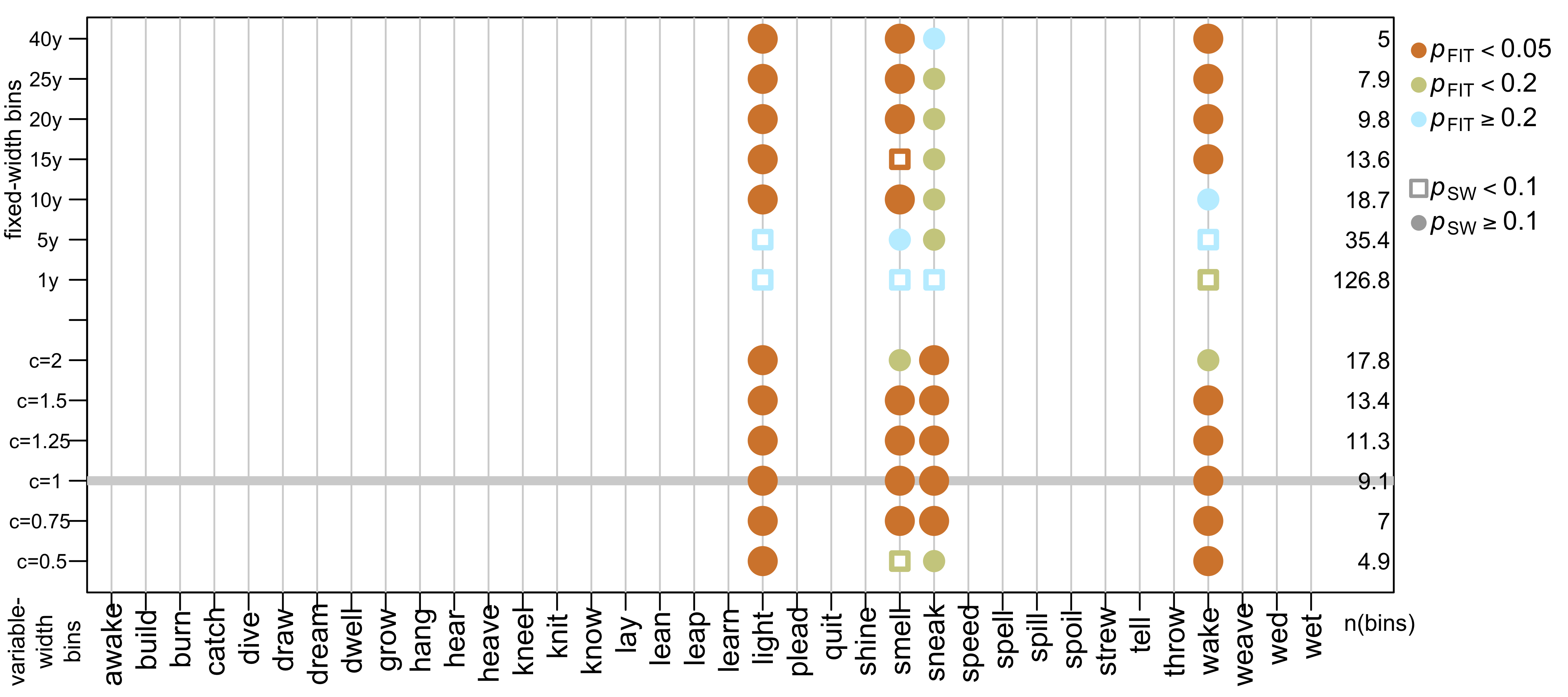<!-- --> --- # Replication of Newberry et al. 2017 (36 verbs) 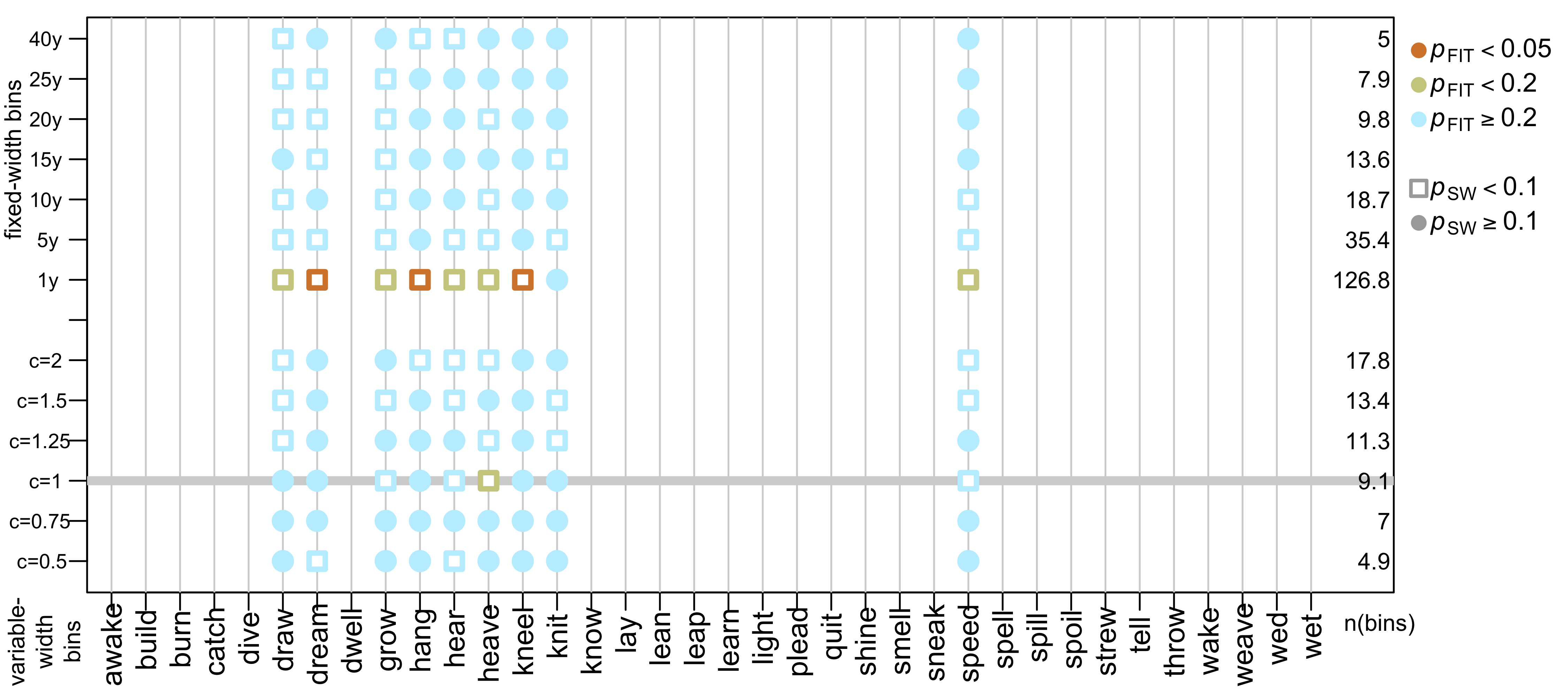<!-- --> --- # Replication of Newberry et al. 2017 (36 verbs) 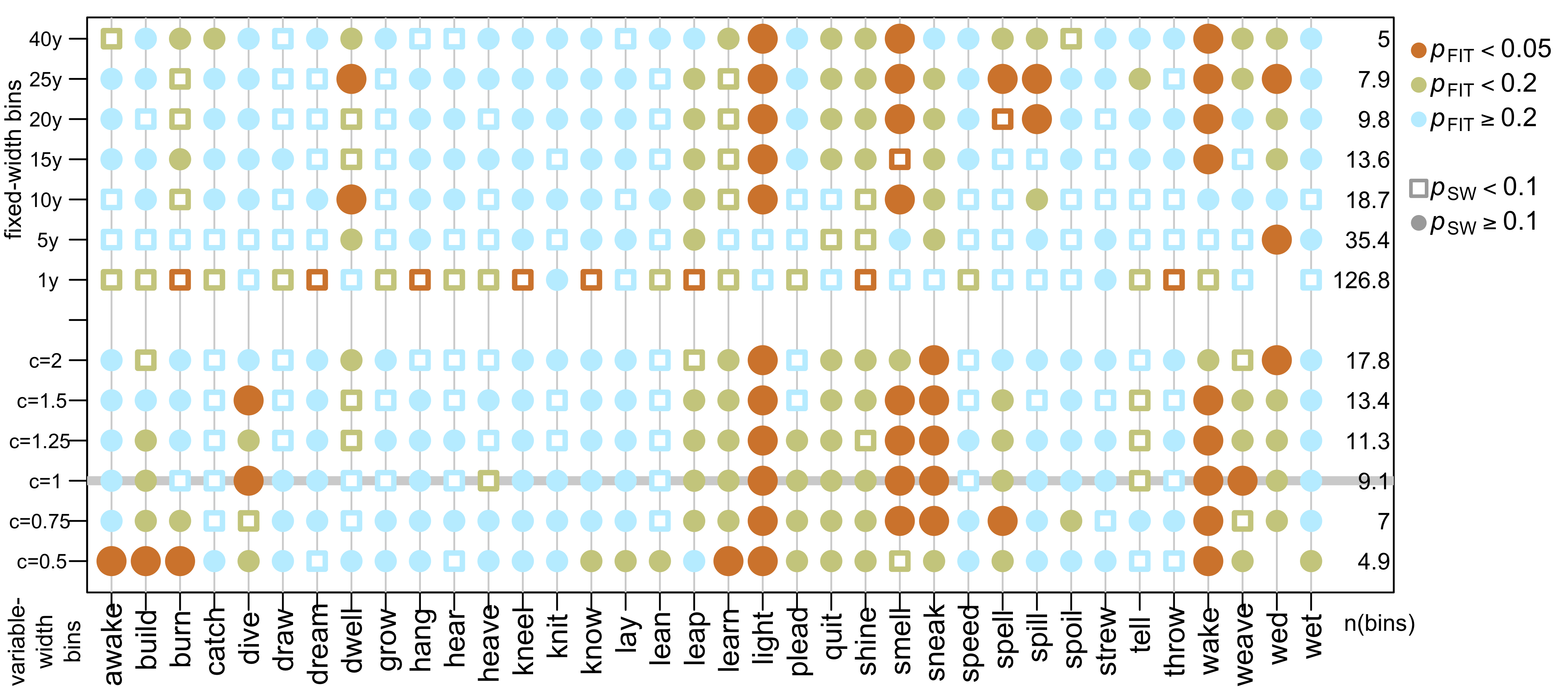<!-- --> --- # Some thoughts -- - In broad strokes, the generalization by Newberry et al. 2017 holds - selection is indeed detected in only ~3..7 verbs (depending on binning), and drift is quite prevalent (at `\(\alpha=0.05\)`). -- - However, for most individual time series, the FIT result varies between binnings (except for ~3 almost unambiguous cases) -- - So is it a good approach to study language change?<br>Depends on the goal. -- - But still, what's the deal with the variation in the results...? --- 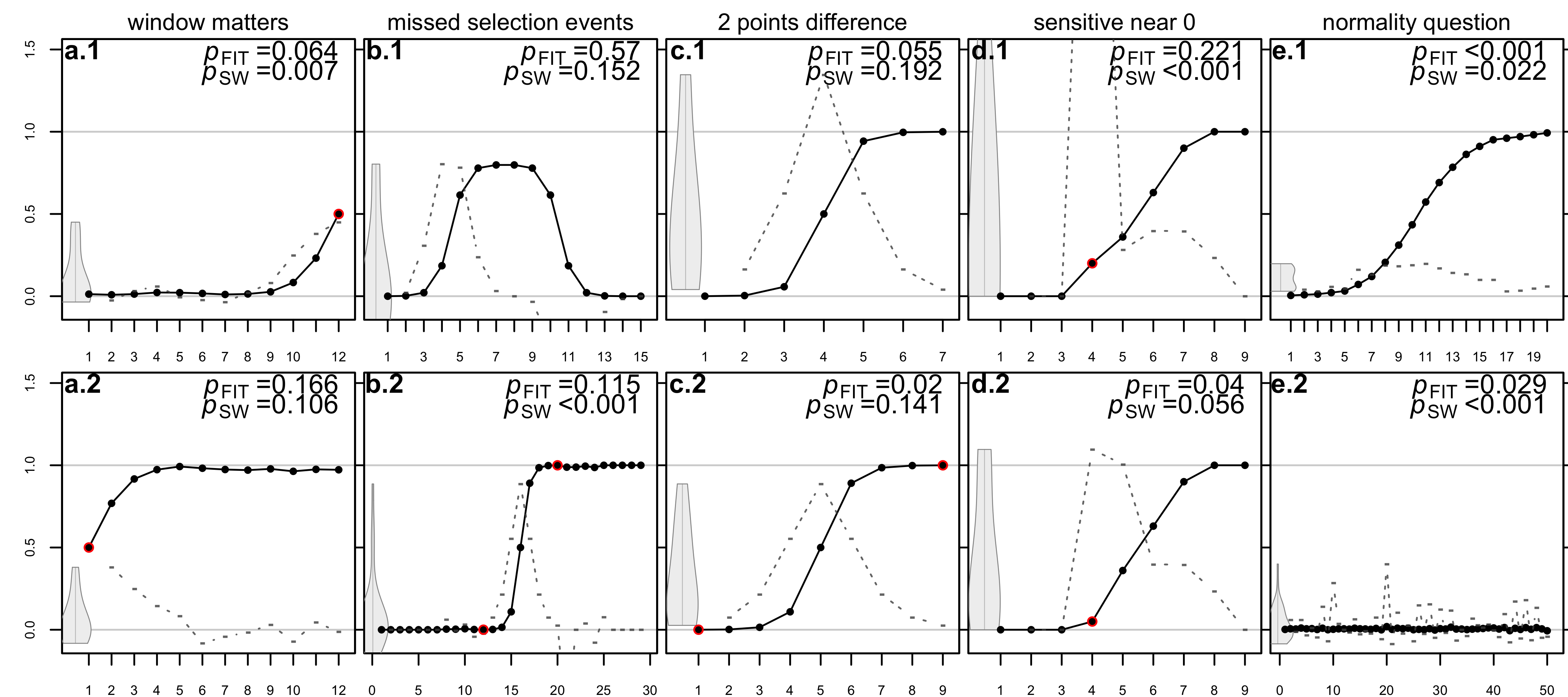<!-- --> .small[What's going on?] --- 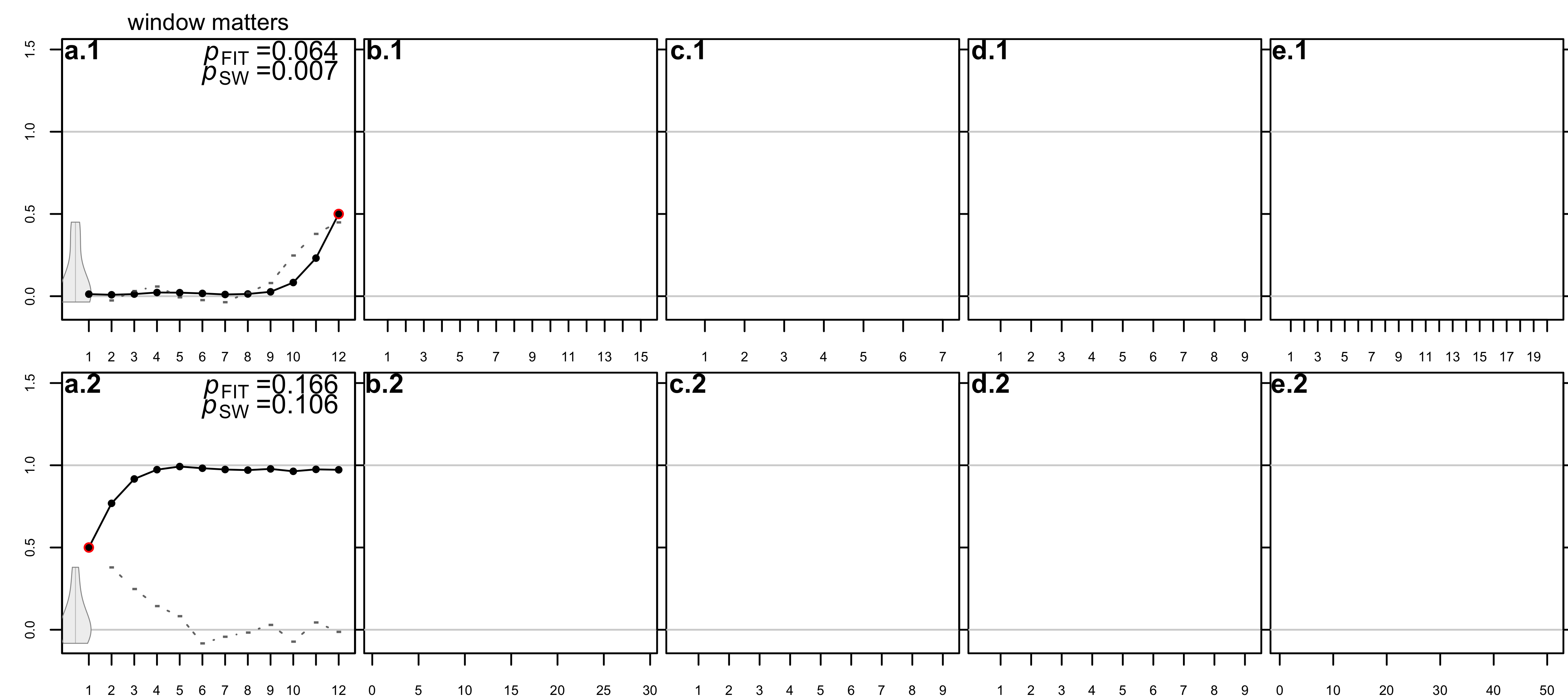<!-- --> .small[(e.g. _spill, burn_)] --- 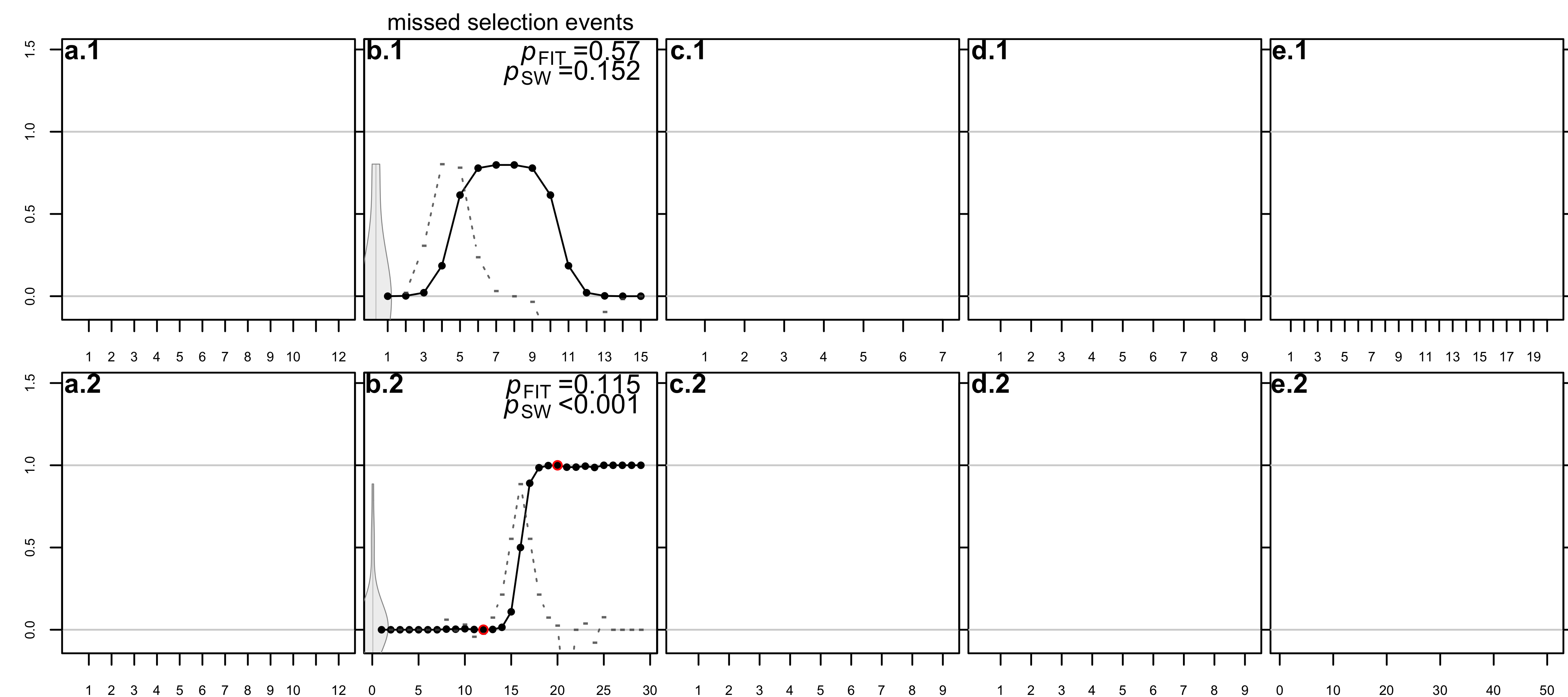<!-- --> .small[(e.g. _knit_)] --- 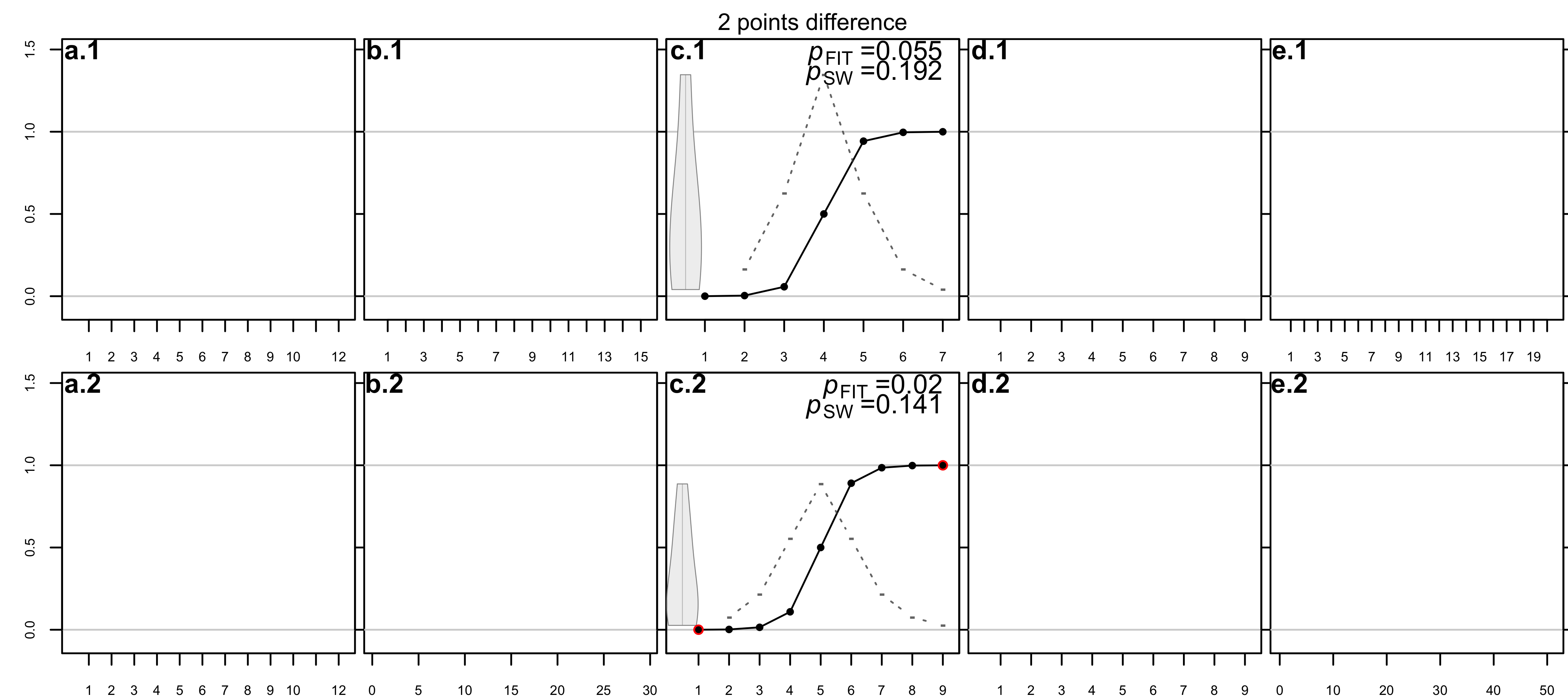<!-- --> .small[(differences between number of bins)] --- 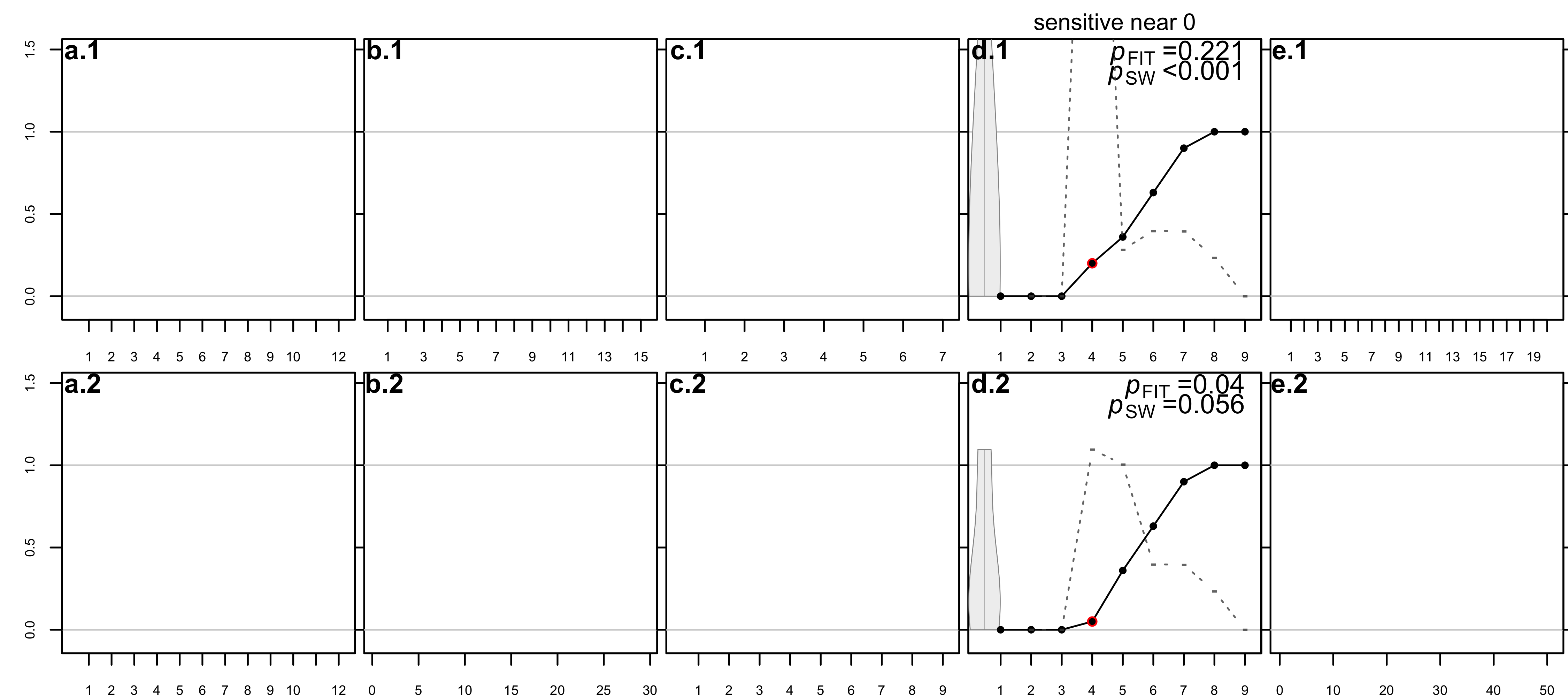<!-- --> --- 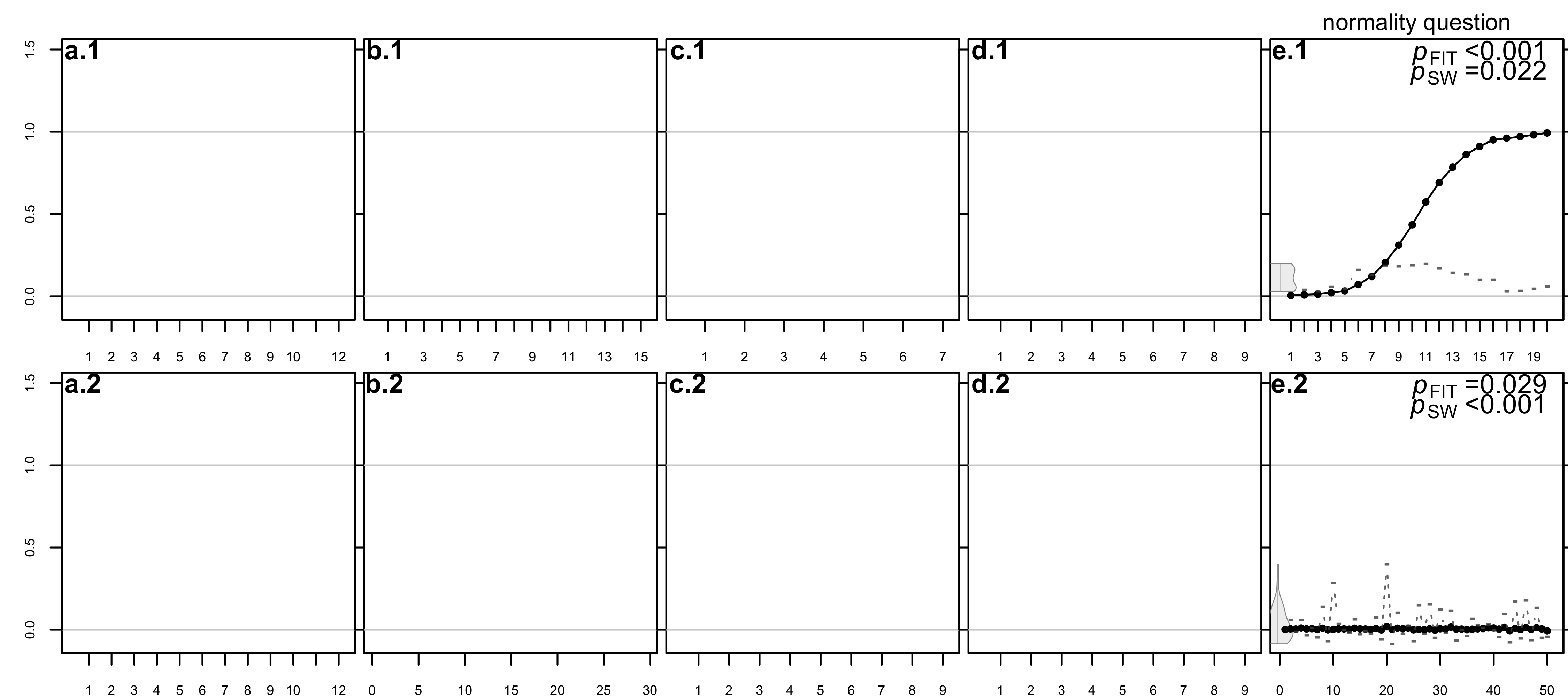<!-- --> .small[(e.g., _tell_)] --- class:inverse # Simulating change and applying binning<br>to determine the reasonable application range<br>of the FIT --- # Simulating change and binning -- - Run a large number of Wright-Fisher simulations with 200 different selection coefficients `\(s \in [0,5]\)` -- - 200 generations, the "mutant" starting at 5% and 50% of the population of size 1000. -- - For each `\(s\)`, bin the series in successively fewer number of bins <br>e.g. 200 (bin length 1) -> 100 (length 2) -> 66 (length 3) etc -- - Repeat every combination 100x for good measure --- .pull-left[
] .pull-right[
] --- 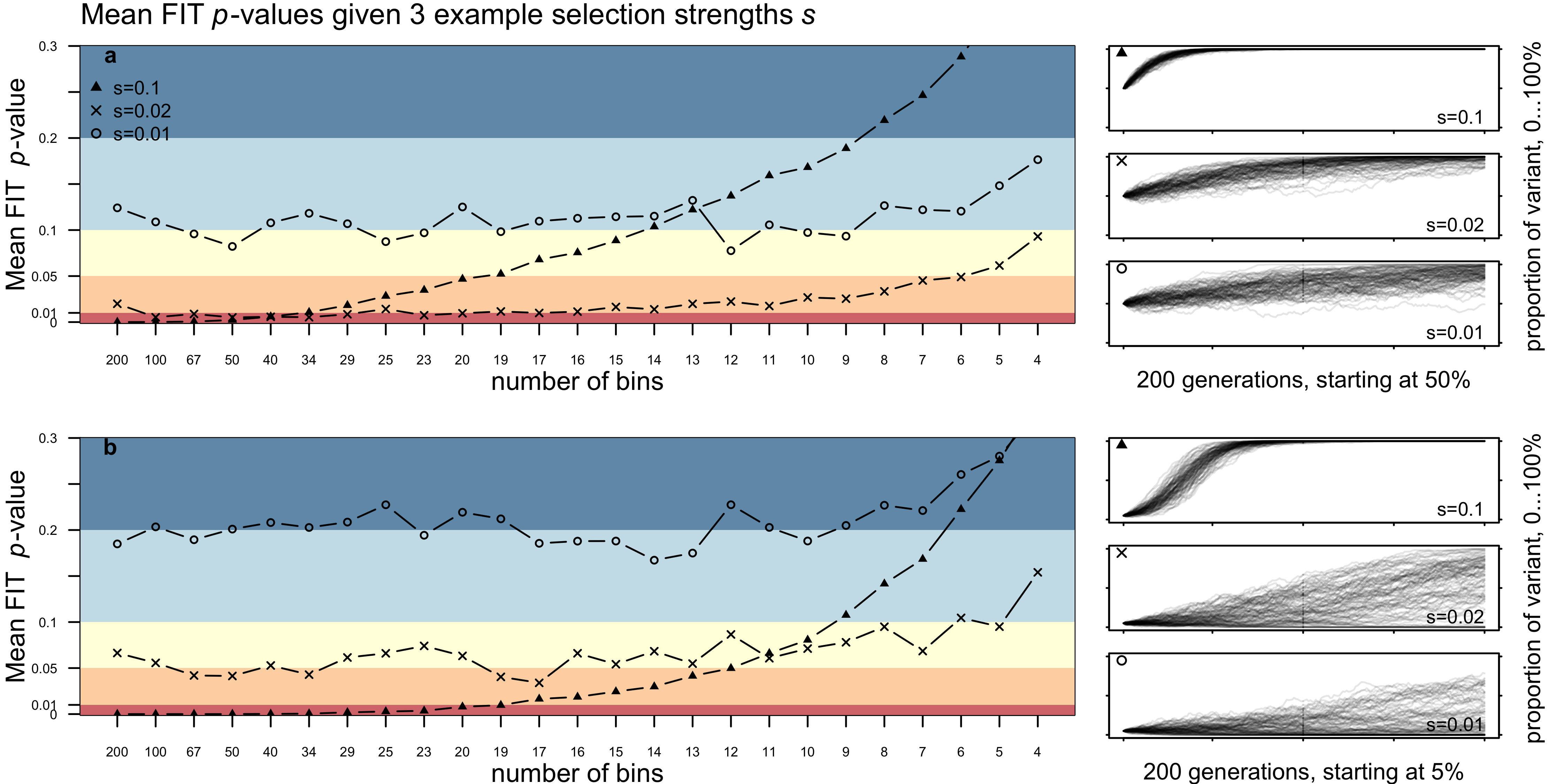<!-- --> --- 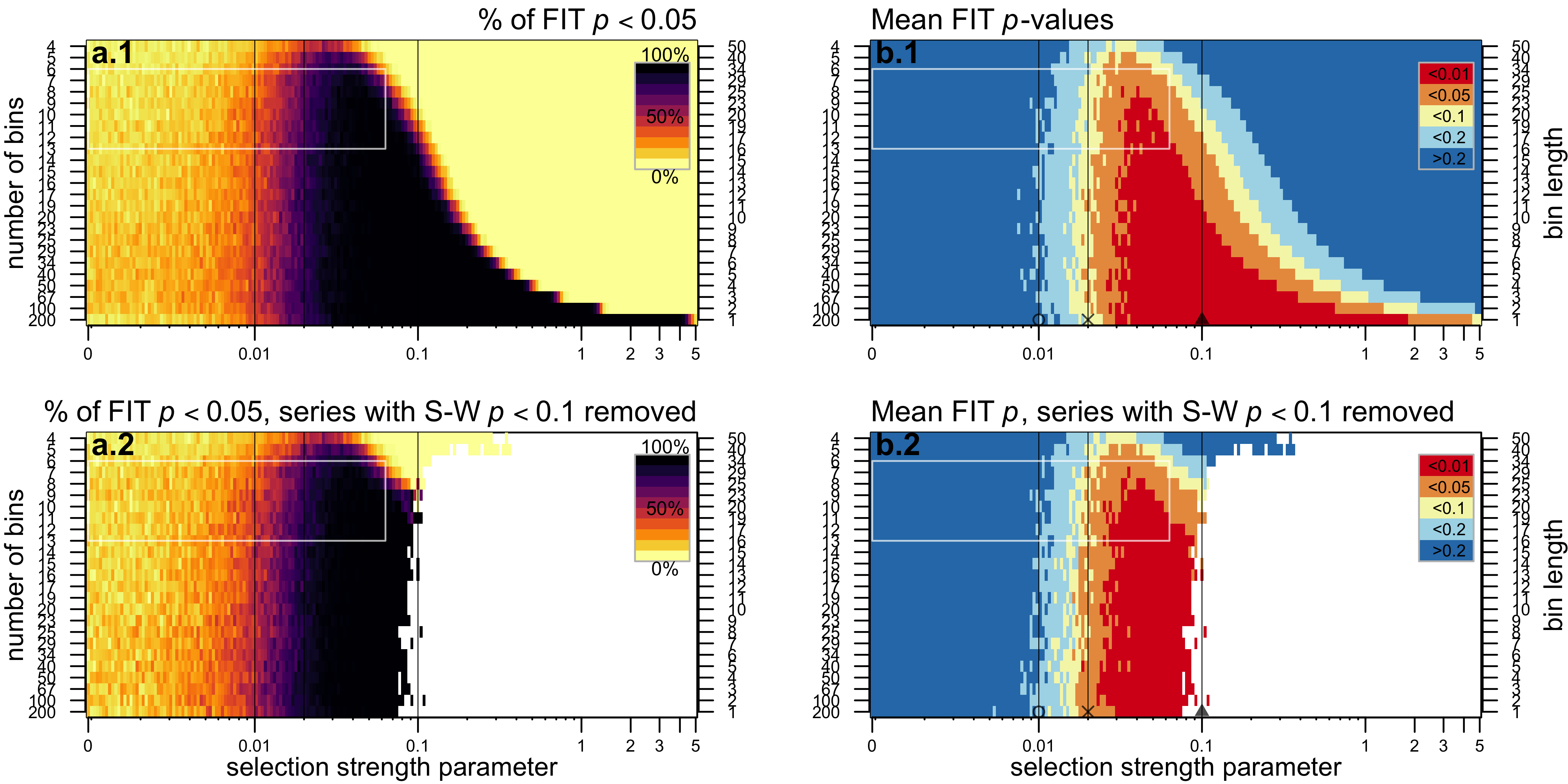<!-- --> .small[(start at 5%)] --- 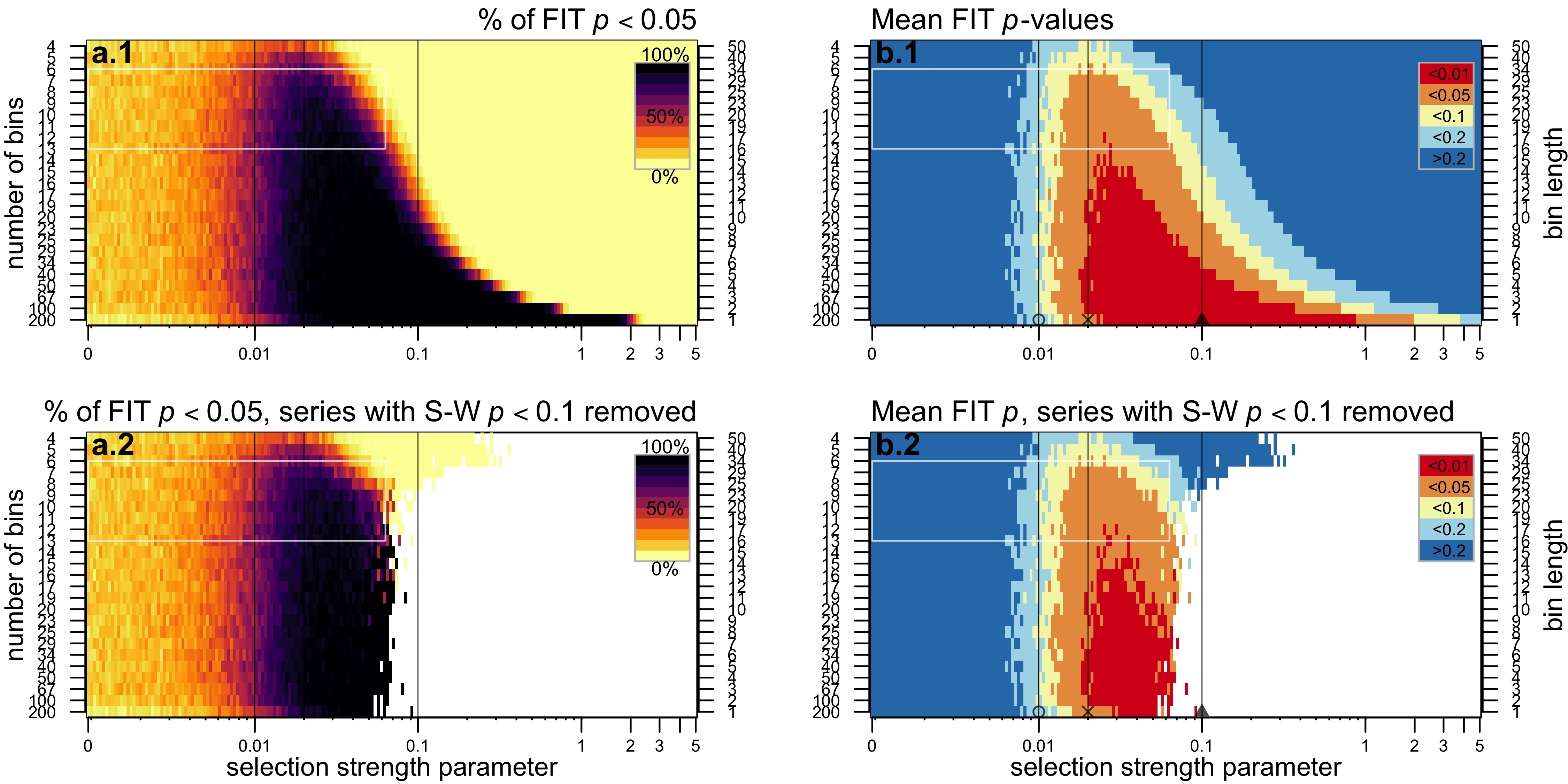<!-- --> .small[(start at 50%)] --- # Observations - The FIT is insensitive to binning when selection is too weak ( `\(s<0.01\)`) to be detected; beyond about `\(s>0.02\)` (depending on the start value) sensitivity to binning increases (false negatives) -- - `\(0.01<s<0.02\)` is relatively insensitive; but also where binning can instead decrease the FIT `\(p\)`-value (false positives) -- - The normality assumption is systematically violated when `\(s\)` approaches 0.1 (unless extreme binning is applied, which increases the false negative rate) --- # Range of applicability of the FIT for linguistic data - Conditions where the FIT is not reliably applicable: - partially completed changes, too short series - too few data points (sensitive to binning & absorption adjustment) - too long series (multiple events or processes) - too high selection (particularly with high binning) - small near-boundary fluctuations (false positives) - steep changes from boundary->non-boundary values - monotonically increasing series (normality assumption) - Where it is: - weak selection, non-monotonic series away from 0/1, but window covering enough of (a single) change --- class: inverse # Conclusions -- - What a time to be alive! .small[(data, methods, tools)] -- - We evaluated the proposal of Newberry et al. 2017<br>Found that the results are dependent on corpus binning, small sample effects, and the specifics of the FIT. - Testing vs generating hypotheses; degrees of freedom -- - Fixing the issues would invite answers to numerous interesting questions --- class: inverse - Fixing these issues would invite answers to numerous interesting questions such as -- - Do different parts of grammar/lexicon experience stronger drift? -- - What is the relationship of selection strength and niche in language change? .small[(cf. Laland2001, Altmann2011)] -- - Can different types of selection (top-down, grassroots, momentum) be distinguished? .small[(Amato2018, Stadler2016)] -- - What is the role of drift in creole evolution? .small[(Strimling2015)] -- - In semantic change? .small[(Hamilton2016)] -- - Are some languages changing more due to drift than others? Relation to community size? .small[(Reali2018, Atkinson2015)<br>(+et-al.'s)] --- class: inverse # Conclusions - What a time to be alive! .small[(data, methods, tools)] - We evaluated the proposal of Newberry et al. 2017<br>Found that the results are dependent corpus binning, small sample effects, and the specifics of the FIT. - Testing vs generating hypotheses; degrees of freedom - Fixing the issues would invite answers to numerous interesting questions - Identifying the role of drift vs selection in language change is an important goal, but: care with applying such tests to linguistic data, to avoid biases due to specifics of the domain and the particular test. - Slides, code & arXiv link at http://andreskarjus.github.io --- class: inverse # Acknowledgements... - Kenny Smith, Richard Blythe, Simon Kirby - Mitchell Newberry - Alison Feder - .small[Support by the Kristjan Jaak program, funded by the Archimedes Foundation & Ministry of Education and Research of Estonia]